The Hidden Costs of Poor Data Quality
Poor data quality impacts an organization’s efficiency and bottom line more profoundly than most realize. Research indicates that data quality issues can cost companies millions of dollars annually. These costs arise not only from correcting data errors but also from missed opportunities, compliance penalties, and reputational harm. At its core, data quality influences the trust organizations have in their data and, subsequently, in the decisions that data informs.
Building a Foundation for Data Quality
A strong data governance framework requires several key foundations. Below are some core principles that support a robust data quality strategy:
Accessibility: Data should be readily accessible across departments. Critical data should not reside in silos, and access to third-party data sources should be seamless. This requires reliable connectivity, as well as monitoring mechanisms to alert teams of access issues.
Accuracy: Data must accurately represent real-world events or entities. Common accuracy issues arise from data entry errors, inconsistent calculations, or unit mismatches, which can lead to costly mistakes.
Completeness: Incomplete data can skew insights and impede decision-making. For example, missing addresses in a customer database can undermine marketing efforts. Ensuring completeness involves identifying essential fields and verifying that critical data points are filled.
Consistency: Data consistency means having uniform values across systems. A “single version of the truth” ensures departments are aligned, reducing the confusion that stems from inconsistent data records.
Precision and Relevance: Data should be recorded with the required level of detail, while outdated information should be regularly pruned. For example, patient data in a healthcare setting must reflect current treatments, while outdated records could have severe consequences for care.
Timeliness: Data should be updated frequently enough to meet business demands. Including timestamps on reports, for example, allows users to identify outdated information, supporting better decision-making.
Observability: Observability provides visibility into the health of data across systems. It enables teams to monitor data quality proactively, addressing issues before they impact operations.
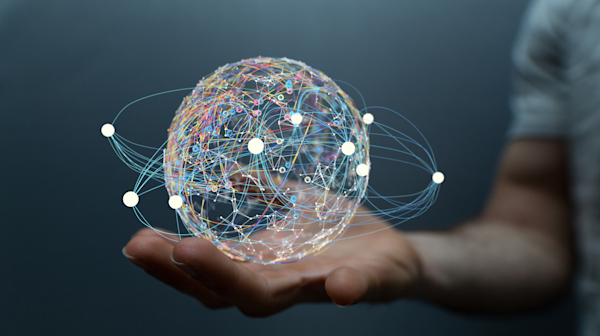
Real-World Impacts of Data Quality Failures
Data quality failures can have serious real-world consequences. Consider, for example, an incident where incorrect credit scores affected loan approvals for thousands of customers, damaging both the financial well-being of those affected and the reputation of the company responsible. Similarly, data errors have led to homes mistakenly being demolished—a stark reminder of how data inaccuracies can disrupt lives. These cases illustrate the far-reaching effects of data quality issues and highlight the importance of robust data practices.
The Path to Improved Data Maturity
Improving data quality requires a clear strategy aligned with the organization’s data maturity level. Organizations generally progress through four levels of data maturity:
Data Neutral: At this foundational level, data quality practices are minimal, reactive, and manual. As data volumes increase, issues become frequent and challenging to manage.
Data Aware: Companies at this stage have started implementing basic data quality processes, with some validation checks and minimal automation. Tools such as Excel or SQL are often used manually to address issues as they arise.
Data Critical: Data quality here becomes proactive, with dedicated governance and KPIs to measure data health. Regular audits and structured processes improve consistency and accuracy.
Data Driven: At the highest level, data is central to strategy, with advanced governance and AI readiness in place. Data quality is thoroughly embedded in the organization, supporting complex analytics and predictive modeling.
Practical Steps for Strengthening Data Quality and Governance
For companies looking to improve their data maturity, implementing a strategic data governance framework can help. Here are some key steps:
Start Small: Address immediate pain points rather than attempting a comprehensive overhaul. Implement a simple governance structure that allows stakeholders to address these areas effectively.
Build Ownership: Each department should own its data quality. Establishing roles like data stewards who take responsibility for data accuracy and completeness in their areas can help build accountability.
Establish Policies and Playbooks: Define policies for managing data issues and create playbooks to guide responses. This can prevent confusion during data quality incidents and ensure a streamlined, efficient approach to problem-solving.
Regular Cadence: Data governance meetings should be a recurring event to ensure data quality remains a priority. Regular engagement keeps data quality practices aligned with evolving business needs.
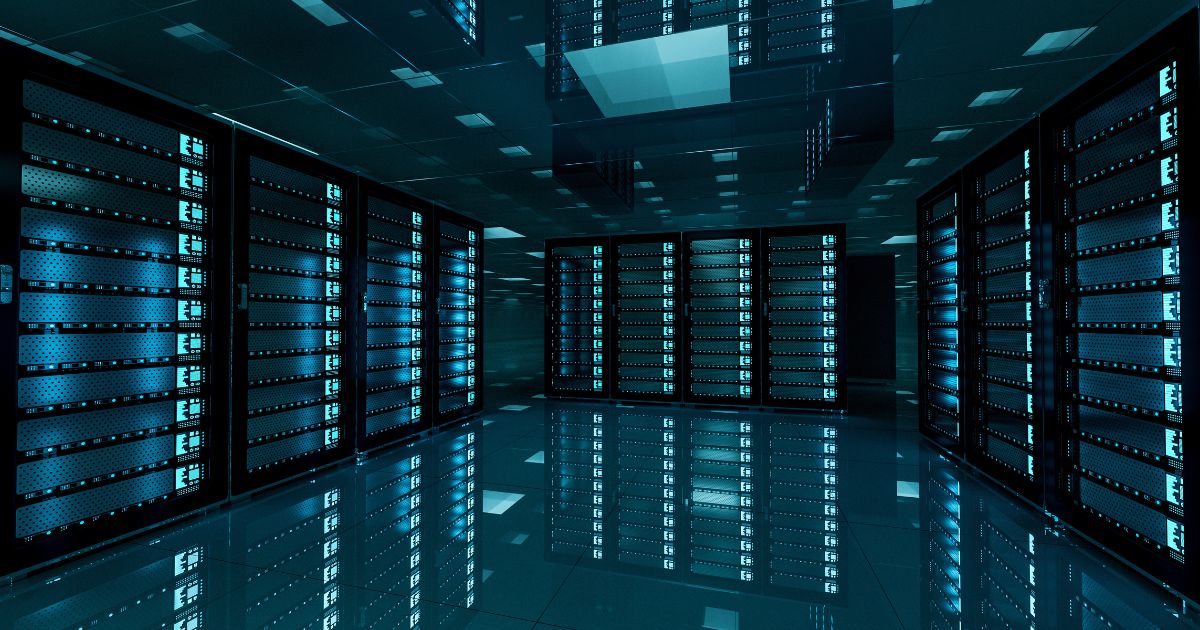
Selecting the Right Tools for Data Quality
Maintaining data quality requires a strong technology stack, particularly as organizations grow and data complexity increases. Below are some essential tools to consider:
Master Data Management (MDM): Tools like Informatica and TIBCO consolidate data to create a single, accurate record across systems. MDM addresses accessibility, consistency, and observability, providing a centralized repository for accurate reference data.
Data Testing and Validation: Platforms like DBT and Validator enable automated testing of data quality, ensuring that data meets organizational standards before reaching end-users. Validation processes are vital for catching issues early and maintaining data reliability.
Data Cataloging and Observability: Solutions such as Microsoft Purview and Monte Carlo provide a high-level view of data assets and their lineage, offering transparency and aiding troubleshooting in complex environments.
Cultivating a Culture of Data Quality
Data quality is as much about culture as it is about technology. Organizations that excel in data management often foster a culture of continuous improvement, similar to Toyota’s Kaizen approach in manufacturing. To instill this culture, organizations must engage leadership and encourage cross-departmental collaboration. By treating data quality as a shared responsibility rather than a siloed function, companies can ensure that data quality practices permeate the entire organization.
Final Thoughts
In today’s data-centric world, poor data quality is a costly risk that can no longer be overlooked. Addressing accessibility, accuracy, completeness, and other foundational aspects of data quality builds trust in data, enabling better decision-making and driving improved business outcomes. By adopting a structured approach to data maturity, investing in the right tools, and creating a culture that values data quality, organizations can turn data into a powerful, reliable asset that fuels sustainable growth and innovation.
If you missed the full presentation, make sure to explore the Tomorrow Technology. Today series for more insights on AI, data ecosystems, and platform engineering.